In the world of advertising technology (AdTech), measuring the effectiveness of campaigns is essential. Marketers are constantly looking for ways to ensure that their advertising spend is translating into actual results, whether it’s sales, leads, or brand awareness. One method that has gained significant attention in recent years is incrementality testing. This technique helps marketers understand the true impact of their advertising efforts by isolating the effect of ads from other factors.
In this article, we’ll explore what incrementality testing is, why it matters, and how it works in the context of AdTech.
What is Incrementality Testing?
Incrementality testing is a method used to determine the actual contribution of an advertising campaign to a specific outcome, such as increased sales or user engagement. It seeks to answer the question: What would have happened if the advertising campaign had not run?
To do this, incrementality testing isolates the impact of the campaign from other external factors. It does so by comparing the behavior of a group exposed to the ads (the treatment group) with a similar group that was not exposed (the control group). This helps marketers understand the real effect of the campaign and avoid overestimating its impact.
Why is Incrementality Testing Important?
1. Accurate Measurement of Ad Impact
Traditional methods of measuring ad performance, like click-through rates (CTR) and impressions, often fail to show the true effectiveness of an ad. For example, a user may click on an ad but not make a purchase. Without proper context, it’s hard to know whether the ad actually influenced their decision.
Incrementality testing provides a more accurate measurement by focusing on whether the ad caused a change in behavior that would not have occurred without it.
2. Optimizing Ad Spend
With incrementality testing, advertisers can determine which channels, creatives, or audiences are driving the most value. By understanding the true impact of each campaign, they can make data-driven decisions about where to allocate their budget. This ensures that advertising dollars are spent more effectively, improving return on investment (ROI).
3. Avoiding False Positives
Sometimes, external factors or events outside of a campaign can influence user behavior. Without incrementality testing, an advertiser may mistakenly attribute a rise in sales or engagement to a particular ad, even if other factors were the real cause. Incrementality testing helps avoid this pitfall by isolating the ad’s effect and providing a clearer picture of its true influence.
4. Improving Long-Term Strategy
Incrementality testing helps advertisers refine their long-term marketing strategies. By understanding how specific campaigns contribute to overall business objectives, marketers can fine-tune their approaches over time, ensuring continuous improvement.
How Does Incrementality Testing Work?
1. Setting Up the Control and Treatment Groups
The first step in incrementality testing is creating two groups: the treatment group (those who are exposed to the ad) and the control group (those who are not). These groups are designed to be as similar as possible in terms of demographics, behavior, and other relevant factors.
2. Running the Campaign
Once the groups are established, the campaign is launched for the treatment group. The control group should remain unaffected by the ad, ensuring that any difference in outcomes can be attributed to the campaign itself.
3. Measuring Outcomes
After the campaign runs for a set period, marketers analyze the behavior of both groups. They look at key metrics, such as sales, app downloads, or website visits, to determine whether the treatment group performed better than the control group. The difference in performance between the two groups represents the incremental impact of the ad.
4. Statistical Analysis
To ensure the results are statistically significant, marketers use statistical methods to analyze the data. This analysis helps them account for any variations between the groups that could influence the outcome, ensuring that the measured effect is truly due to the ad and not other external factors.
Types of Incrementality Tests
There are several approaches to conducting incrementality tests, each suited to different advertising goals:
1. A/B Testing
A/B testing is indeed one of the simplest forms of incrementality testing. It involves running two versions of an ad (or no ad at all) and then comparing the performance between the two groups. This test is often used to measure the effectiveness of different creatives, copy, or targeting strategies.
2. Geo-Testing
Geo-testing involves running the same campaign in different geographical regions and then comparing the results. A company might expose one region to the ad, while using the other as the control group. Companies with location-based campaigns often use this approach to isolate the impact of advertising on regional sales or foot traffic.
3. Matched Market Testing
In this approach, marketers select two similar markets (e.g., two different cities or online platforms). They expose one market to the ad campaign, while keeping the other as the control. The difference in performance between these markets shows the incremental effect of the campaign.
4. Media Mix Modeling (MMM)
MMM involves analyzing historical data across multiple channels and then applying statistical methods to determine the impact of each channel. Although not a direct comparison of treatment and control groups, it helps advertisers understand the long-term incremental effect of different advertising channels on sales or other business outcomes.
Challenges in Incrementality Testing
1. Data Collection
For incrementality testing to work effectively, the right data needs to be collected. This can be challenging, especially when dealing with complex ad campaigns or cross-channel marketing efforts. Ensuring that the control and treatment groups are as similar as possible indeed requires robust data analysis.
2. External Factors
Even with careful testing, external factors such as seasonality, economic conditions, or competitor actions can influence the results. Marketers need to account for these factors when analyzing the results of incrementality tests to ensure accurate conclusions.
3. Cost and Time
Running controlled tests, particularly large-scale experiments, can be costly and time-consuming. Advertisers need to weigh the potential benefits of incrementality testing against the investment required to run the tests.
Conclusion
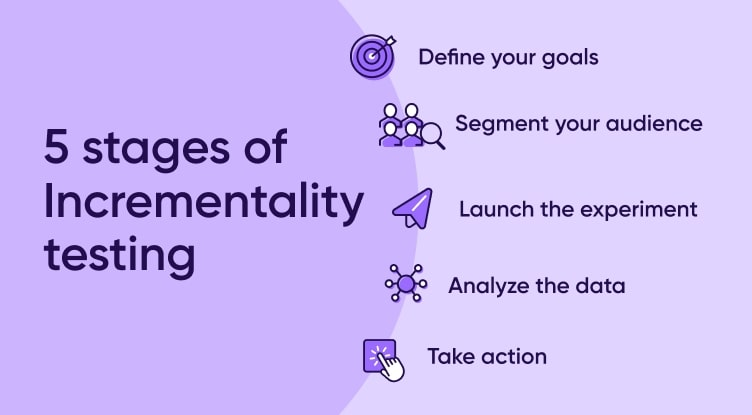
Incrementality testing is indeed a powerful tool for advertisers seeking to measure the true impact of their campaigns. By isolating the effect of ads and comparing them to a control group, marketers can gain a clearer understanding of what works and also what doesn’t. Although challenges exist, incrementality testing provides insights that can lead to more effective campaigns, better budget allocation, and improved ROI in the competitive world of AdTech.
Frequently Asked Questions (FAQs)
1. What is incrementality testing in AdTech?
AdTech uses incrementality testing to measure the true impact of an advertising campaign by comparing the behavior of a group exposed to the ad (treatment group) with a group not exposed (control group). This method helps isolate the effect of the ad from other factors.
2. Why is incrementality testing important for advertisers?
It provides a more accurate measurement of ad effectiveness, thus allowing advertisers to understand the true contribution of their campaigns. This helps optimize ad spend, avoid overestimating results, and further improve long-term marketing strategies.
3. What are the different types of incrementality tests?
Common types include A/B testing, geo-testing, matched market testing, as well as media mix modeling. Each method helps measure the incremental effect of ads across different environments or regions.
4. What challenges do marketers face with incrementality testing?
Challenges include collecting accurate data, accounting for external factors like seasonality or competitor actions, as well as the cost and time required to run large-scale tests. However, these challenges are outweighed by the valuable insights provided.